Dual Memory Network Model for Biased Product Review Classification
Yunfei Long*, Mingyu Derek Ma*, Qin Lu, Rong Xiang, Chu-Ren Huang
(* Equal contribution)
Oct 31, 2018
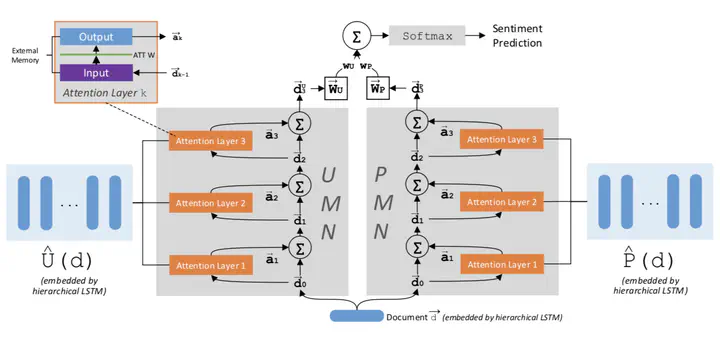
Abstract
In sentiment analysis (SA) of product reviews, both user and product information are proven to be useful. Current tasks handle user pro- file and product information in a unified model which may not be able to learn salient fea- tures of users and products effectively. In this work, we propose a dual user and product memory network (DUPMN) model to learn user profiles and product reviews using separate memory networks. Then, the two repre- sentations are used jointly for sentiment pre- diction. The use of separate models aims to capture user profiles and product information more effectively. Compared to state-of-the-art unified prediction models, the evaluations on three benchmark datasets, IMDB, Yelp13, and Yelp14, show that our dual learning model gives performance gain of 0.6%, 1.2%, and 0.9%, respectively. The improvements are also deemed very significant measured by p-values.
Type
Publication
In 9th Workshop on Computational Approaches to Subjectivity, Sentiment and Social Media Analysis, Brussels, Belgium.